Crucial Problems on Heaps for Successful Interviews
Written on May 28, 2023
Views : Loading...
1. Find the Median of a Stream:
Given a continuous stream of numbers, design a data structure that efficiently finds the median of the numbers seen so far.
Algorithm:
- Initialize an empty max-heap (lowerHeap) to store the smaller half of the numbers and an empty min-heap (higherHeap) to store the larger half of the numbers.
- Whenever a new number is received from the stream:
- If both heaps are empty, add the number to the max-heap.
- If the number is smaller than the median (the root of the max-heap), add it to the max-heap; otherwise, add it to the min-heap.
- After adding a number, ensure that the heaps are balanced, with the size difference between them at most 1. If the difference exceeds 1, perform the following steps:
- If the max-heap (lowerHeap) has more elements, remove the root element (the maximum) from the max-heap and add it to the min-heap.
- If the min-heap (higherHeap) has more elements, remove the root element (the minimum) from the min-heap and add it to the max-heap.
- To find the median, check the sizes of the heaps:
- If the heaps have the same size, the median is the average of the roots of both heaps.
- If the max-heap (lowerHeap) has more elements, the median is the root of the max-heap.
- If the min-heap (higherHeap) has more elements, the median is the root of the min-heap.
import heapq
class MedianFinder:
def __init__(self):
self.lowerHeap = [] # max-heap
self.higherHeap = [] # min-heap
def addNum(self, num):
if len(self.lowerHeap) == len(self.higherHeap):
# Add the number to the max-heap (lowerHeap) and negate it to simulate a max-heap behavior
heapq.heappush(self.lowerHeap, -num)
else:
# Add the number to the min-heap (higherHeap)
heapq.heappush(self.higherHeap, num)
# Balance the heaps if necessary
if self.higherHeap and -self.lowerHeap[0] > self.higherHeap[0]:
# Swap the root elements of both heaps
heapq.heappush(self.higherHeap, -heapq.heappop(self.lowerHeap))
heapq.heappush(self.lowerHeap, -heapq.heappop(self.higherHeap))
def findMedian(self):
if len(self.lowerHeap) == len(self.higherHeap):
# Both heaps have the same size, so the median is the average of the roots
return (-self.lowerHeap[0] + self.higherHeap[0]) / 2.0
else:
# Max-heap (lowerHeap) has more elements, so the median is the root of the max-heap
return -self.lowerHeap[0]
2. Sliding Window Median:
Given an array of integers and a window size, find the median of each window as it slides through the array.
Algorithm:
-
Define two data structures, a max-heap (also known as a max-priority queue) and a min-heap (min-priority queue). The max-heap will store the smaller half of the numbers in the window, while the min-heap will store the larger half.
-
Initialize the heaps and create a window of the given size. Insert the first k elements of the array into the heaps. If the window size is odd, insert the first k/2 + 1 elements into the max-heap and the rest into the min-heap. If the window size is even, insert the first k/2 elements into both heaps.
-
For each subsequent window, do the following:
- Remove the element that is going out of the window from the heaps.
- Insert the new element coming into the window into the appropriate heap.
- Balance the heaps by ensuring that the difference in the number of elements between the max-heap and min-heap is at most 1. If the difference becomes larger, move the root element of the larger heap to the other heap.
-
Find the median for each window:
- If the window size is odd, the median is the root element of the max-heap.
- If the window size is even, the median is the average of the root elements of both heaps.
-
Repeat step 3 and step 4 until you have processed all windows in the array.
void insert(int val, multiset<int>&low, multiset<int>&high,int &k){
int a = *low.rbegin(); // current median
if (a<val){
high.insert(val);
if (high.size()>k/2){
low.insert(*high.begin());
high.erase(high.find(*high.begin()));
}
} else {
low.insert(val);
if (low.size()>(k+1)/2){
high.insert(*low.rbegin());
low.erase(low.find(*low.rbegin()));
}
}
}
void erase(int val, multiset<int>&low, multiset<int>&high){
if (high.find(val) != high.end()) high.erase(high.find(val));
else low.erase(low.find(val));
if (low.empty()) {
low.insert(*high.begin());
high.erase(high.find(*high.begin()));
}
}
signed main()
{
multiset<int>low,high;
int n,k;
cin>>n>>k;
vector < int > a(n,0);
for (int i = 0; i < n; i++) cin>>a[i];
low.insert(a[0]);
for (int i = 1; i < k; i++) insert(a[i],low,high,k);
cout<<*low.rbegin()<<" ";
for (int i = k; i < n; i++) {
if (k==1){
insert(a[i],low,high,k);
erase(a[i-1],low,high);
}
else{
erase(a[i-k],low,high);
insert(a[i],low,high,k);
}
cout<<*low.rbegin()<<" ";
}
cout<<endl;
return 0;
}
3.Kth Smallest Element in a Sorted Matrix:
Given a sorted matrix of integers, find the Kth smallest element.
- Binary Search Time Complexity : $O(K log N)$
- Bucket Sort Time Complexity: $O(n)$
- Heap Time Complexity: $O(N log K)$
Algorithm:
- Initialize a min-heap data structure.
- Iterate over the first row of the matrix and insert each element into the min-heap along with its corresponding row and column indices.
- Start a loop that runs K times:
- Extract the minimum element (smallest) from the min-heap. This element will be the Kth smallest element so far.
- Check if there is an element in the next column of the current row. If so, insert it into the min-heap.
- Increment the row index and insert the element from the next row in the same column into the min-heap.
- After the loop finishes, the extracted element from the min-heap will be the Kth smallest element.
import heapq
def kthSmallest(matrix, k):
if not matrix or not matrix[0]:
return None
rows, cols = len(matrix), len(matrix[0])
min_heap = []
# Insert elements from the first row into the min-heap
for j in range(cols):
heapq.heappush(min_heap, (matrix[0][j], 0, j))
# Perform K iterations
for _ in range(k):
element, row, col = heapq.heappop(min_heap)
# If there is a valid element in the next column of the current row, insert it into the min-heap
if col + 1 < cols:
heapq.heappush(min_heap, (matrix[row][col + 1], row, col + 1))
# If it's not the last row, insert the element from the next row in the same column
if row + 1 < rows:
heapq.heappush(min_heap, (matrix[row + 1][col], row + 1, col))
return element
4. Find K Pairs with Smallest Sums:
Given two sorted arrays, find the K pairs with the smallest sums.
Required Time Complexity : $O(k * log(min(k, n)))$
Algorithm:
- Create a min-heap data structure to store the pairs based on their sums. In Python, you can use the heapq module for this purpose.
- Initialize an empty min-heap and push the first k pairs into the heap, sorted by their sums. You can compute the sums of the pairs and push them onto the heap with the pair itself.
- Iterate through the remaining pairs, starting from index k. For each pair, calculate its sum and compare it with the root of the min-heap (the pair with the smallest sum so far).
- If the sum is smaller than the root's sum, remove the root from the heap and push the current pair onto the heap.
- Continue this process until you have processed all pairs. At the end, the k pairs with the smallest sums will be stored in the min-heap.
- Extract the k pairs from the min-heap in ascending order of their sums. You can use the heappop function to remove the root of the heap repeatedly until you have extracted k pairs.
import heapq
def kSmallestPairs(nums1, nums2, k):
if not nums1 or not nums2:
return []
heap = []
for i in range(min(k, len(nums1))):
heapq.heappush(heap, (nums1[i] + nums2[0], i, 0))
pairs = []
while k > 0 and heap:
_, i, j = heapq.heappop(heap)
pairs.append([nums1[i], nums2[j]])
if j + 1 < len(nums2):
heapq.heappush(heap, (nums1[i] + nums2[j+1], i, j+1))
k -= 1
return pairs
5. Minimum Cost to Hire K Workers:
Given a list of workers with their respective qualities and minimum wage expectations, find the minimum cost to hire K workers such that the ratio of their total quality to total wage is minimized.
This problem is a variant of the classical "Minimum Cost Maximum Flow" problem. This problem can be solved using a combination of sorting and a priority queue.
Algorithm:
- Create a list of workers, each containing their quality and minimum wage expectations.
- Sort the workers in ascending order based on their wage/quality ratio. This step ensures that the workers with the lowest ratio will be considered first.
- Initialize variables:
- Initialize a priority queue to store the top K workers based on their qualities.
- Initialize a variable to track the sum of the qualities of the workers in the priority queue.
- Traverse the sorted list of workers:
- For each worker, calculate their wage/quality ratio.
- Add the worker to the priority queue.
- Add their quality to the sum of qualities.
- If the priority queue size exceeds K, remove the worker with the highest quality from the queue and subtract their quality from the sum.
- Calculate the total cost by multiplying the sum of qualities by the ratio of the last worker removed from the priority queue.
- Return the total cost, which represents the minimum cost to hire K workers while minimizing the ratio of their total quality to total wage.
import heapq
def mincostToHireWorkers(qualities, wages, K):
workers = sorted([(q, w) for q, w in zip(qualities, wages)], key=lambda x: x[1]/x[0])
heap = []
sum_qualities = 0
min_cost = float('inf')
for q, w in workers:
heapq.heappush(heap, -q)
sum_qualities += q
if len(heap) > K:
sum_qualities += heapq.heappop(heap)
if len(heap) == K:
min_cost = min(min_cost, sum_qualities * w / q)
return min_cost
Open Problem : Find Median in a Large File
This problem statement might not be complete
Given a large file containing a sorted list of integers that cannot fit into memory and are split into chunks, find the median of the numbers efficiently.
Kindly Contact me to contribute a solution.
Other Problems :
- Huffman coding.
- Check if a binary tree is a heap.
Share this blog
Related Posts
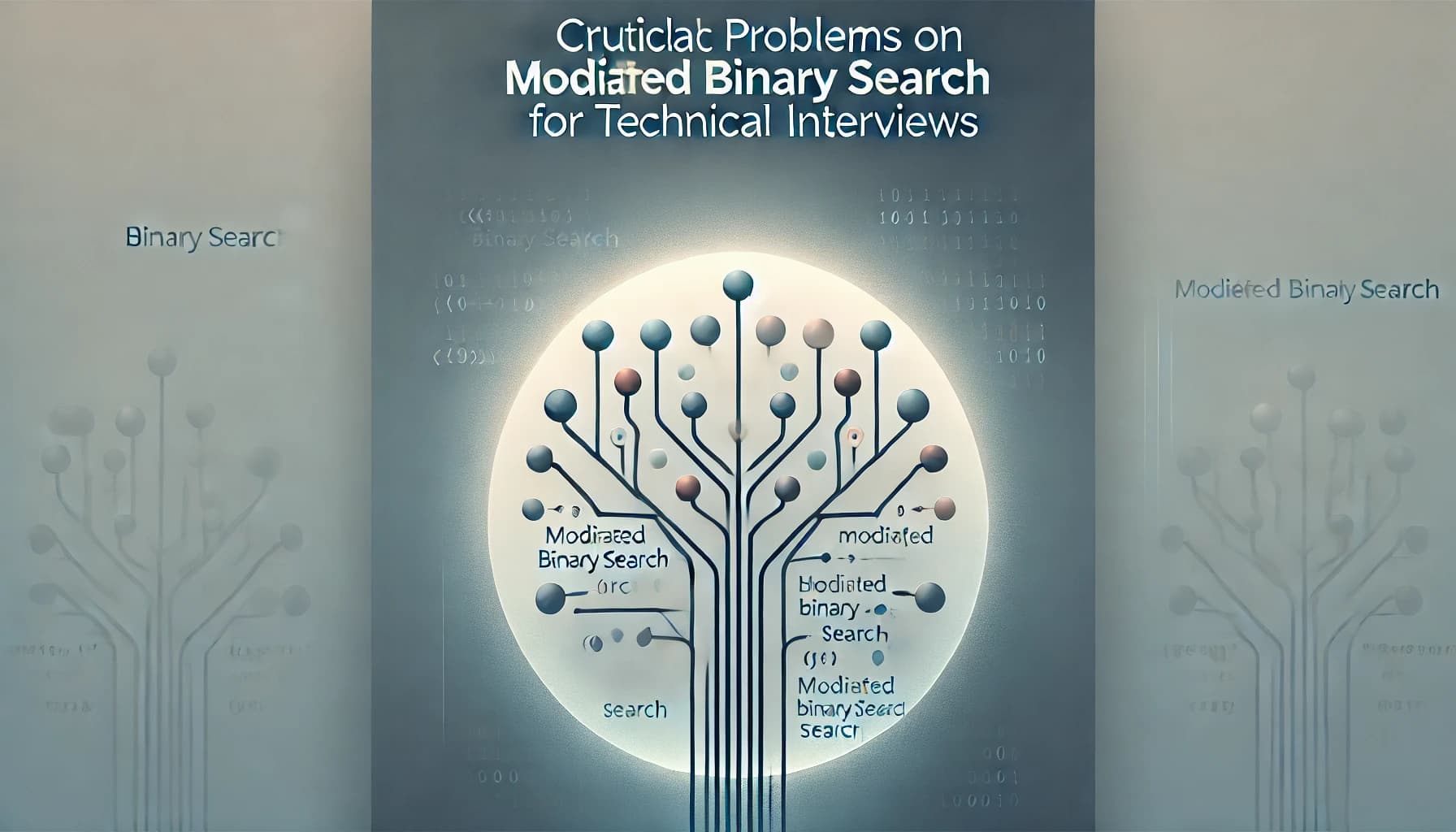
28-05-2023
Discover important problems related to Modified Binary Search that commonly arise in technical inter...
27-05-2023
Explore the essential challenges of sliding window methodologies in this comprehensive blog. Gain va...
26-05-2023
Unlock the potential of the Two Pointer Approach with this comprehensive guide. Explore a curated se...
25-11-2024
In the realm of graph theory, understanding the connectivity of a network is crucial for numerous ap...
24-11-2024
Dive deep into the Z Algorithm, a fundamental technique in string processing. This blog elucidates t...
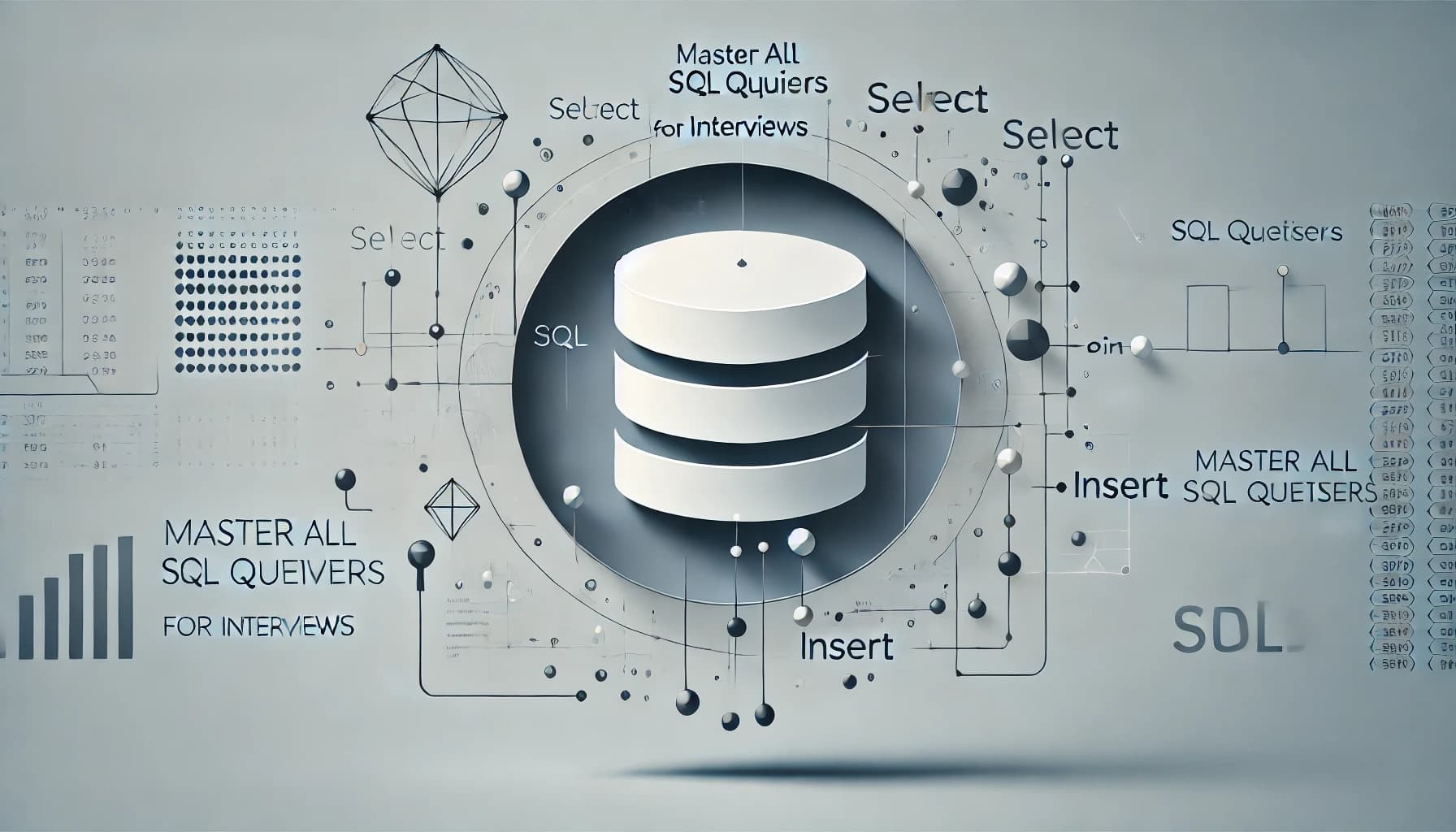
28-07-2024
In this comprehensive guide, you'll master essential SQL queries to ace your database-related interv...
04-11-2023
Explore the most efficient Sorting Algorithms for Competitive Programming and Interviews. Learn abou...
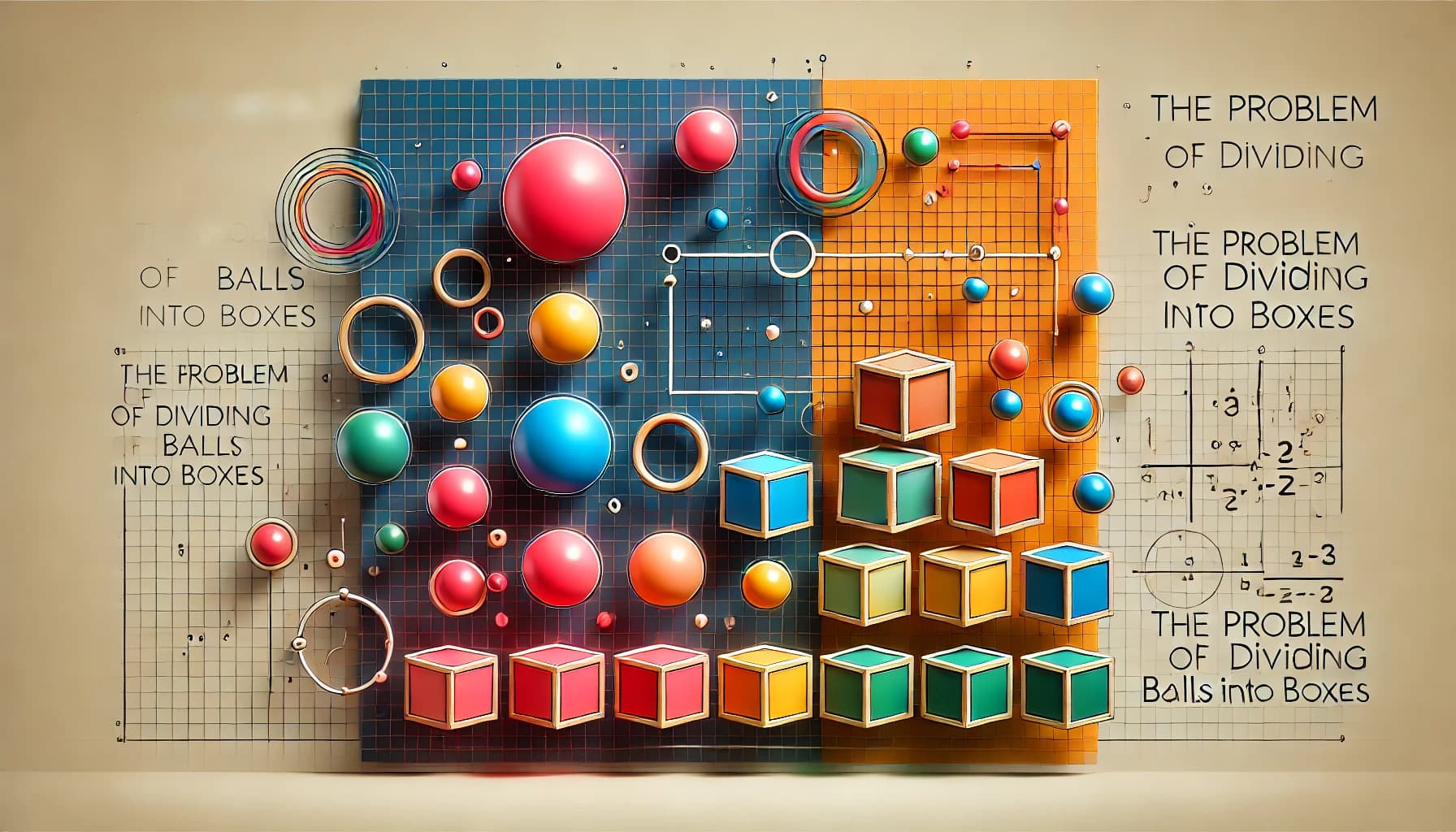
23-07-2023
This document discusses various variants of the problem of dividing n balls into *m* boxes. Markdown...
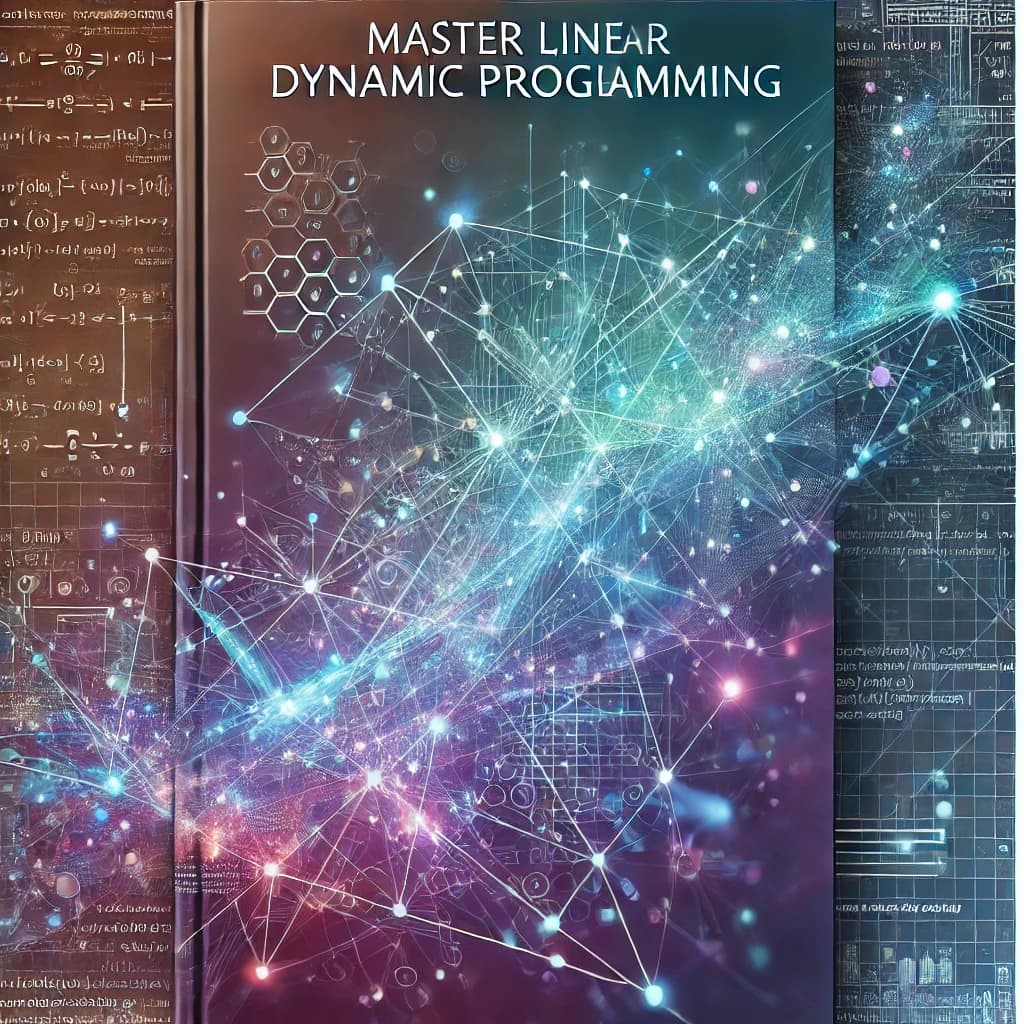
22-07-2023
Enhance your dynamic programming skills with our comprehensive collection of Linear DP problems from...