Z Algorithm and Implementation for String Searching
Written on November 24, 2024
Views : Loading...
Introduction
String searching is a fundamental problem in computer science with applications ranging from text processing to bioinformatics. Among the various algorithms developed to solve this problem efficiently, the Z Algorithm stands out due to its linear time complexity and versatility. In this article, we'll delve into the Z Algorithm, understand its problem statement with illustrative examples, explore a naive implementation, and then walk through the optimized version step-by-step.
Problem Statement
Given a string $ S $ of length $ N $, the goal is to compute an array $ Z $ of length $ N $, where each $ Z[i] $ represents the length of the Longest Common Prefix (LCP) between the string $ S $ and its suffix starting at position $ i $. Formally,
$$ Z[i] = \text{LCP}(S, S.\text{substr}(i)) $$
Examples
Let's consider a few examples to clarify the problem:
-
Example 1:
- Input: $ S = \text{"abcbcba"} $
- Output: $ Z = [7, 0, 0, 0, 0, 0, 1] $
Explanation:
- $ Z[0] = 7 $ (the entire string matches itself)
- $ Z[1] = 0 $ (no common prefix between "abcbcba" and "bcbcba")
- ...
- $ Z[6] = 1 $ (common prefix "a" between "abcbcba" and "a")
-
Example 2:
- Input: $ S = \text{"mississippi"} $
- Output: $ Z = [11, 0, 0, 0, 0, 0, 0, 0, 0, 0, 0] $
Explanation:
- $ Z[0] = 11 $
- All other $ Z[i] = 0 $ since no other suffix shares a prefix with $ S $.
-
Example 3:
- Input: $ S = \text{"ababacaca"} $
- Output: $ Z = [9, 0, 3, 0, 1, 0, 1, 0, 1] $
Explanation:
- $ Z[0] = 9 $
- $ Z[2] = 3 $ (common prefix "aba" between "ababacaca" and "abacaca")
- $ Z[4] = 1 $ (common prefix "a" between "ababacaca" and "acaca")
- And so on.
-
Example 4:
- Input: $ S = \text{"aaaaa"} $
- Output: $ Z = [5, 4, 3, 2, 1] $
Explanation:
- Each suffix shares a progressively shorter prefix with $ S $.
Naive Approach
A straightforward method to compute the $ Z $-array is to iterate over each position in the string and compute the LCP between $ S $ and $ S.\text{substr}(i) $ character by character. Here's how this naive approach can be implemented:
/*
* Author: rishabhxchoudhary
* Created: Saturday, 23.11.2024 09:40 AM (GMT+5:30)
*/
#include <bits/stdc++.h>
using namespace std;
#define int long long int
#define double long double
#define endl '\n'
const int MOD = 1000000007;
signed main()
{
ios_base::sync_with_stdio(0);
cin.tie(0);cout.tie(0);
string s; cin>>s;
int n = s.length();
vector<int>z(n);
for(int i=0;i<n;i++) {
while ( i+z[i] < n && s[z[i]] == s[i+z[i]] ){
z[i]++;
};
}
for(int i=0;i<n;i++) {
cout<<z[i]<<" ";
}
return 0;
}
Analysis of the Naive Approach
- Time Complexity: $ O(N^2) $ in the worst case. For each position $ i $, we might compare up to $ N - i $ characters.
- Space Complexity: $ O(N) $ for storing the $ Z $-array.
While this approach is simple, its quadratic time complexity makes it inefficient for large strings.
Optimized Z Algorithm
The Z Algorithm optimizes the naive approach to run in linear time $ O(N) $ by avoiding redundant comparisons. The key idea is to maintain a window $[L, R)$ (also known as the Z-box) that represents the interval of the string where the substring matches the prefix.
Here's the optimized implementation:
/*
* Author: rishabhxchoudhary
* Created: Saturday, 23.11.2024 09:40 AM (GMT+5:30)
*/
#include <bits/stdc++.h>
using namespace std;
#define int long long int
#define double long double
#define endl '\n'
const int MOD = 1000000007;
signed main()
{
ios_base::sync_with_stdio(0);
cin.tie(0);cout.tie(0);
string s; cin>>s;
int n = s.length();
vector<int>z(n);
z[0] = n;
int l = 0, r = 0;
for(int i = 1; i < n; i++) {
if(i < r) {
z[i] = min(r - i, z[i - l]);
}
while(i + z[i] < n && s[z[i]] == s[i + z[i]]) {
z[i]++;
}
if(i + z[i] > r) {
l = i;
r = i + z[i];
}
}
for(int i=0;i<n;i++) {
cout<<z[i]<<" ";
}
return 0;
}
Step-by-Step Optimizations
Let's dissect the optimized code and understand the enhancements made over the naive approach.
1. Initializing the Z-array
vector<int> z(n);
z[0] = n;
- Explanation: The first element $ Z[0] $ is always equal to the length of the string $ N $, as the entire string matches itself.
2. Introducing the Z-box
int l = 0, r = 0;
- Explanation: We maintain a window $[L, R)$ that denotes the interval where the substring matches the prefix. Initially, this window is empty.
3. Iterating Through the String
for(int i = 1; i < n; i++) {
// ...
}
- Explanation: We start from the second character (index 1) since $ Z[0] $ is already defined.
4. Case When $ i $ Lies Within the Current Z-box
if(i < r) {
z[i] = min(r - i, z[i - l]);
}
-
Explanation:
-
Scenario: If the current position $ i $ is within the existing Z-box $[L, R)$, we can leverage previously computed $ Z $-values to avoid redundant comparisons.
-
Calculation:
- $ Z[i] $ is at least the minimum of:
- The remaining length of the Z-box: $ R - i $
- The previously computed $ Z $-value: $ Z[i - L] $
- $ Z[i] $ is at least the minimum of:
-
Mathematically:
$$ Z[i] = \min(R - i, Z[i - L]) $$
-
Rationale: If $ Z[i - L] $ is less than $ R - i $, it means the substring starting at $ i $ matches the prefix for $ Z[i - L] $ characters, and we can safely assign this value. Otherwise, the match extends beyond the current Z-box, and we need to perform further comparisons.
-
5. Extending the Z-box
while(i + z[i] < n && s[z[i]] == s[i + z[i]]) {
z[i]++;
}
-
Explanation:
-
Purpose: After the initial assignment, we attempt to extend the Z-box as far as possible by comparing characters.
-
Process: Increment $ Z[i] $ as long as the characters match and we haven't exceeded the string's length.
-
6. Updating the Z-box Boundaries
if(i + z[i] > r) {
l = i;
r = i + z[i];
}
-
Explanation:
-
Condition: If the current Z-box can be extended beyond the previous $ R $, we update $ L $ and $ R $ to the new boundaries.
-
Mathematical Update:
$$ L = i $$ $$ R = i + Z[i] $$
-
Rationale: This ensures that the window $[L, R)$ always represents the farthest-reaching Z-box encountered so far.
-
Why Does This Optimization Work?
The core idea is to utilize previously computed $ Z $-values to minimize the number of character comparisons. By maintaining the Z-box, we keep track of a window where the substring matches the prefix, allowing us to infer information about other positions within this window.
Example Walkthrough
Let's walk through an example to illustrate how the optimized Z Algorithm works.
Consider the string: $ S = \text{"ababa"} $
Step-by-Step Execution:
-
Initialization:
$$ Z = [5, 0, 0, 0, 0] $$
$ L = 0, R = 0 $
-
Iteration for $ i = 1 $:
- $ i < R $ is false since $ 1 < 0 $ is false.
- Compare $ S[0] $ and $ S[1] $: 'a' vs 'b' → no match.
- $ Z[1] = 0 $
- $ R $ remains $ 0 $.
-
Iteration for $ i = 2 $:
- $ i < R $ is false since $ 2 < 0 $ is false.
- Compare $ S[0] $ and $ S[2] $: 'a' vs 'a' → match.
- Compare $ S[1] $ and $ S[3] $: 'b' vs 'b' → match.
- Compare $ S[2] $ and $ S[4] $: 'a' vs 'a' → match.
- $ Z[2] = 3 $
- Update $ L = 2, R = 5 $
-
Iteration for $ i = 3 $:
- $ i < R $ is true since $ 3 < 5 $.
- $ Z[3] = \min(5 - 3, Z[1]) = \min(2, 0) = 0 $
- Compare $ S[0] $ and $ S[3] $: 'a' vs 'b' → no match.
- $ Z[3] = 0 $
-
Iteration for $ i = 4 $:
- $ i < R $ is true since $ 4 < 5 $.
- $ Z[4] = \min(5 - 4, Z[2]) = \min(1, 3) = 1 $
- Compare $ S[1] $ and $ S[5] $: Out of bounds.
- $ Z[4] = 1 $
- Update $ L = 4, R = 5 $
Final $ Z $-array:
$$ Z = [5, 0, 3, 0, 1] $$
Time and Space Complexity
-
Time Complexity: $ O(N) $
The algorithm processes each character of the string at most twice—once during the initial iteration and potentially once more during the extension of the Z-box.
-
Space Complexity: $ O(N) $
We use an additional array $ Z $ of size $ N $ to store the computed values.
Conclusion
The Z Algorithm is a powerful tool for string processing, offering linear time complexity and efficient computation of the Longest Common Prefix array. By intelligently maintaining a Z-box and leveraging previously computed values, it avoids redundant comparisons inherent in the naive approach. Understanding and implementing the Z Algorithm can significantly optimize solutions for various string-related problems in competitive programming and real-world applications.
Share this blog
Related Posts
25-11-2024
In the realm of graph theory, understanding the connectivity of a network is crucial for numerous ap...
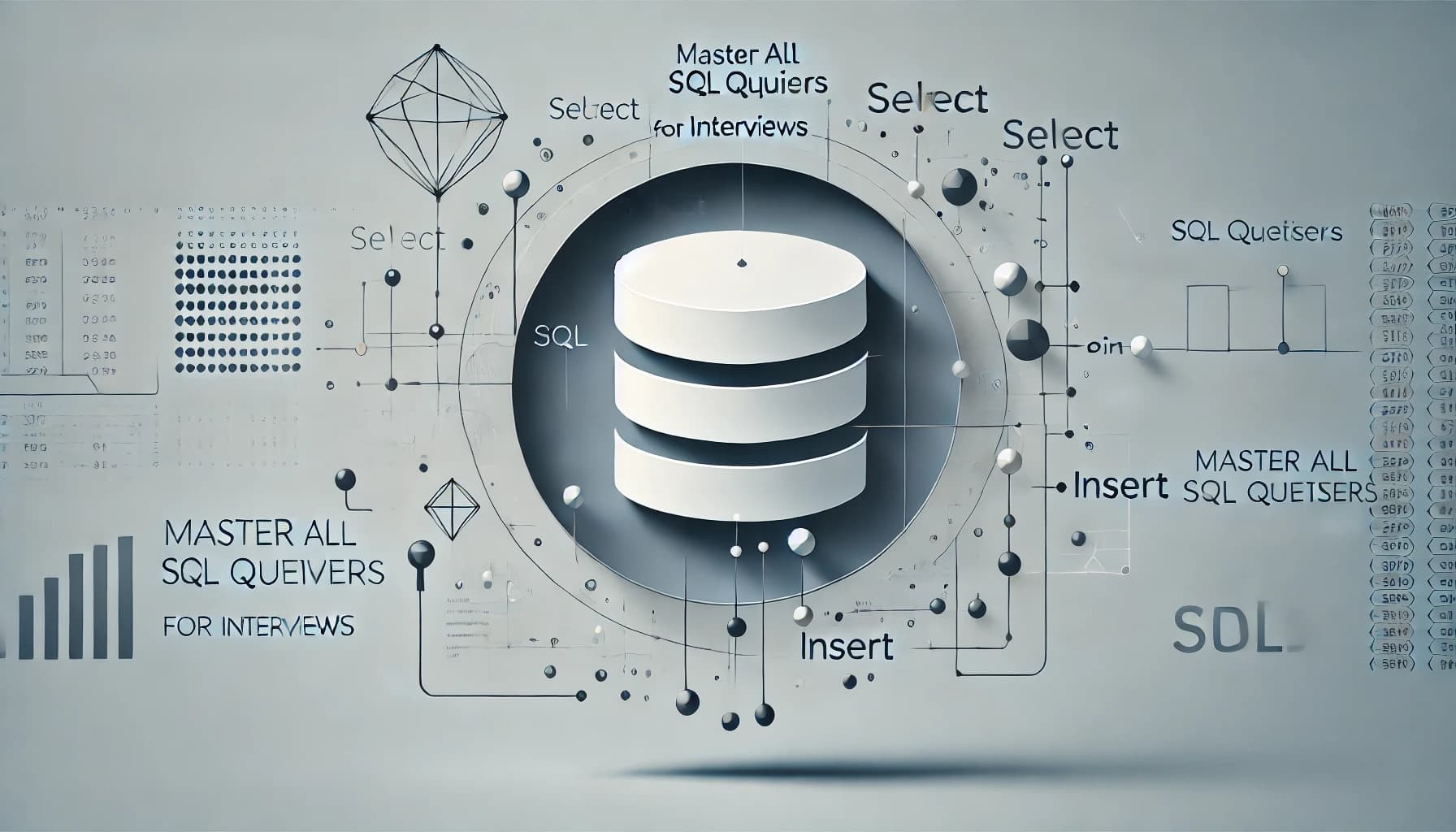
28-07-2024
In this comprehensive guide, you'll master essential SQL queries to ace your database-related interv...
04-11-2023
Explore the most efficient Sorting Algorithms for Competitive Programming and Interviews. Learn abou...
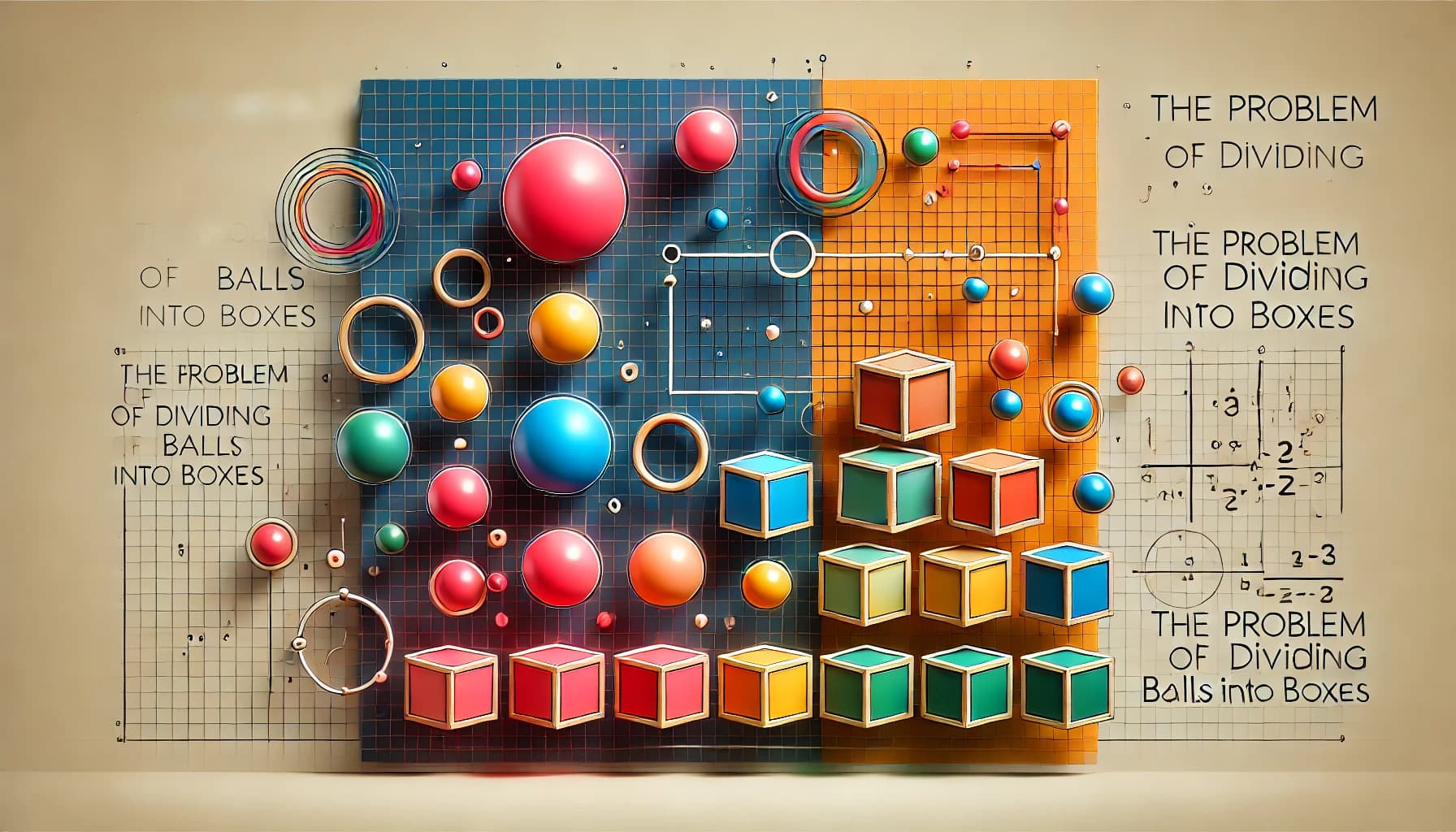
23-07-2023
This document discusses various variants of the problem of dividing n balls into *m* boxes. Markdown...
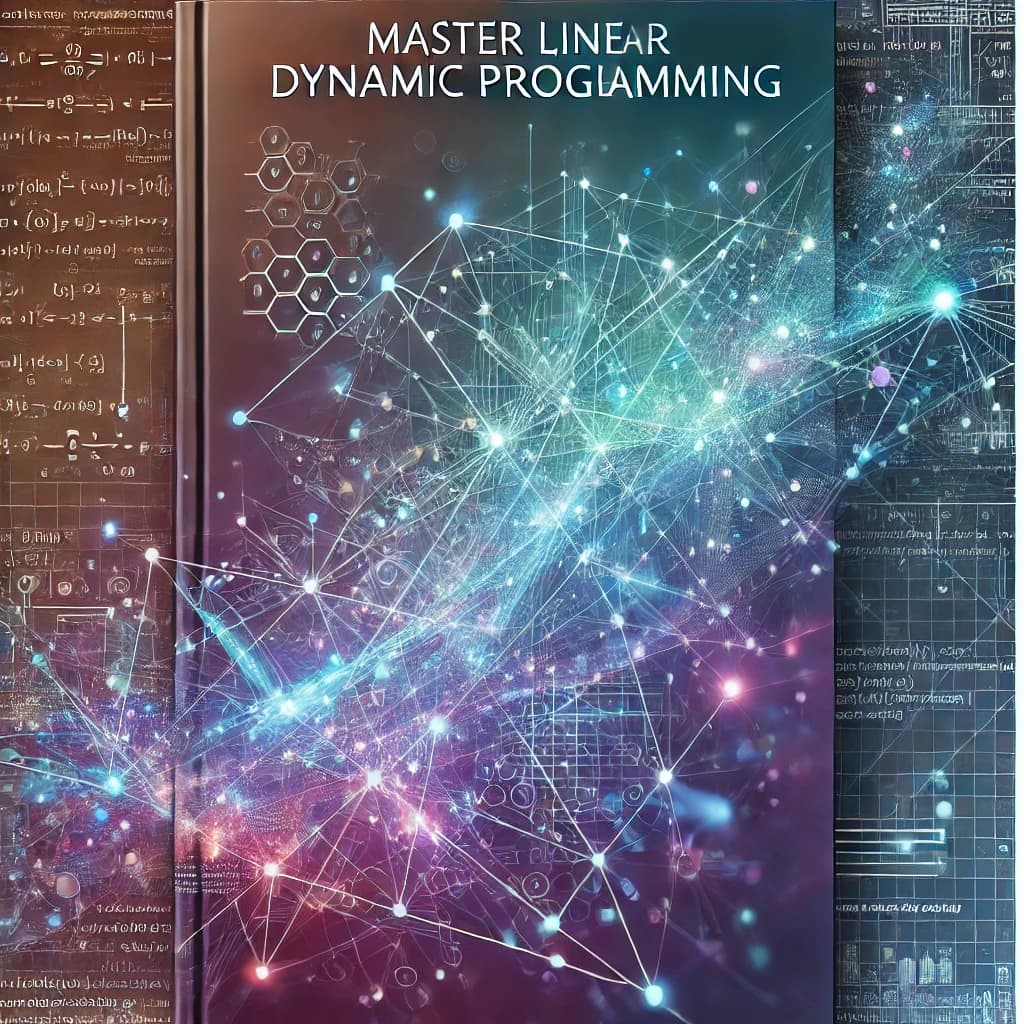
22-07-2023
Enhance your dynamic programming skills with our comprehensive collection of Linear DP problems from...
19-07-2023
Become a Tree Algorithms expert with our comprehensive solutions for CSES and various problem sets. ...
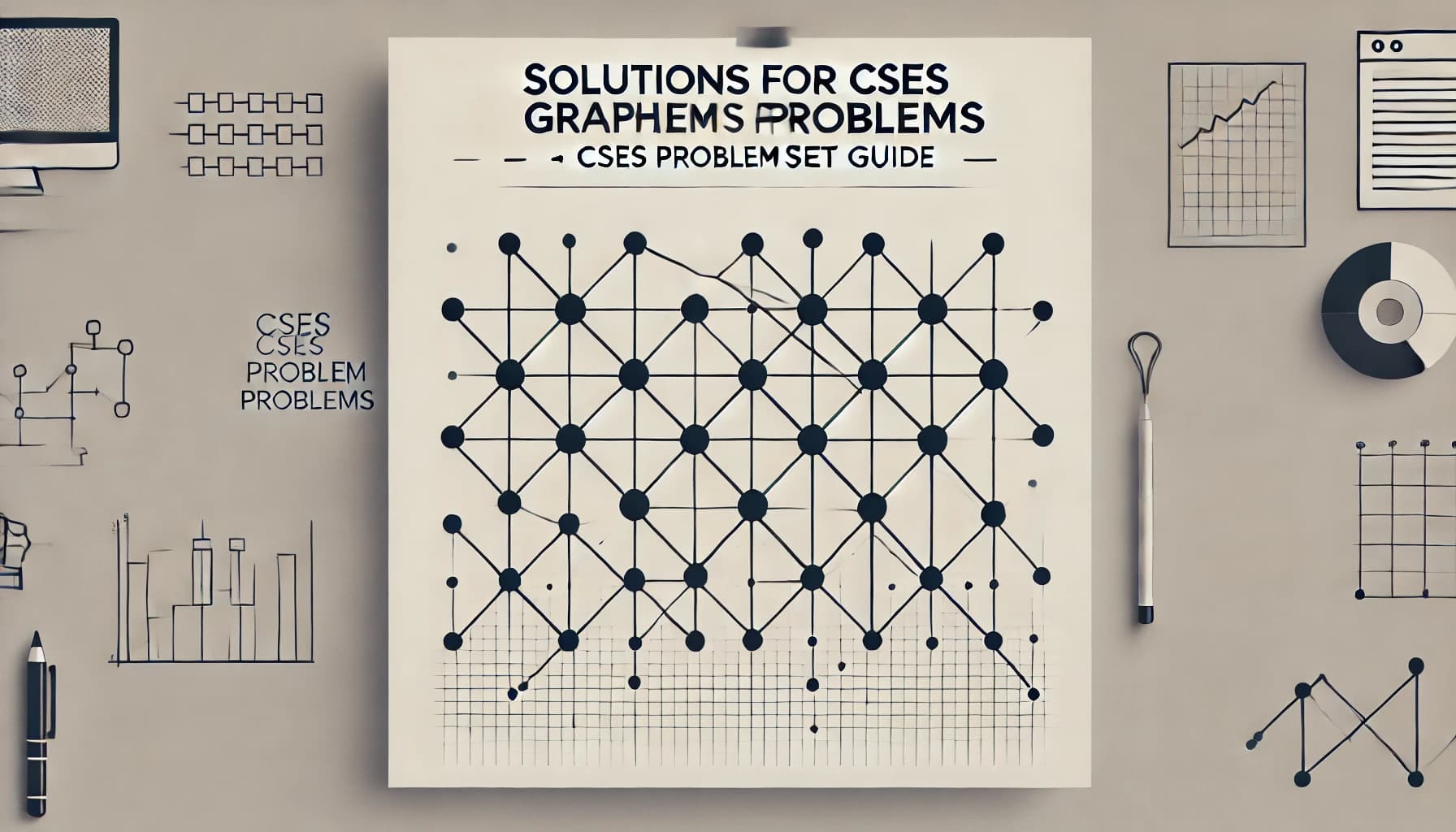
17-07-2023
Explore efficient solutions and master essential algorithms for CSES Graph problems in the CSES Prob...
15-07-2023
Explore efficient solutions to the Sorting and Searching section in the CSES Problem Set. Master ess...
12-07-2023
Explore efficient solutions to dynamic programming problems from the CSES problem set and other repu...